It has never been easier to build artificial intelligence or machine learning-based systems than it is today. The access we now have to massive amounts of computational power and open-source tools is unprecedented. What used to be the exclusive domain of data scientists and systems engineers in academia and research institutes is now accessible to anyone with a laptop and an afternoon to spare.
Artificial intelligence in its most rudimentary definition is the simulation of human intelligence and thinking processes by machines. What constitutes human intelligence and how we define that is another matter altogether. However, this field of study is growing at an alarming pace and has widely varying applications from language processing to automation and art creation. With each passing day we are still discovering new fields in which it can be utilised.

This brings us to an interesting point which is most often the unsung hero of how AI gets about doing what it does, and that is data. Lots of data. This data is usually collected, curated, annotated and organised into datasets which are then used to train machine learning systems. The creation of these datasets is an incredibly involved process, time consuming and meticulous. Unfortunately, the artificial intelligence systems are as effective as the datasets that are used as their building blocks and currently there is a disproportionate amount of data collected from European sources.
The reasons for this are many. Firstly, collecting data is an expensive exercise from a financial standpoint and requires a lot of resources. Most countries have research centers that are dedicated to these tasks and these are sorely lacking in Africa and only exist in certain hotspot countries. Secondly, most datasets are proprietary and as a result inaccessible to most practitioners.
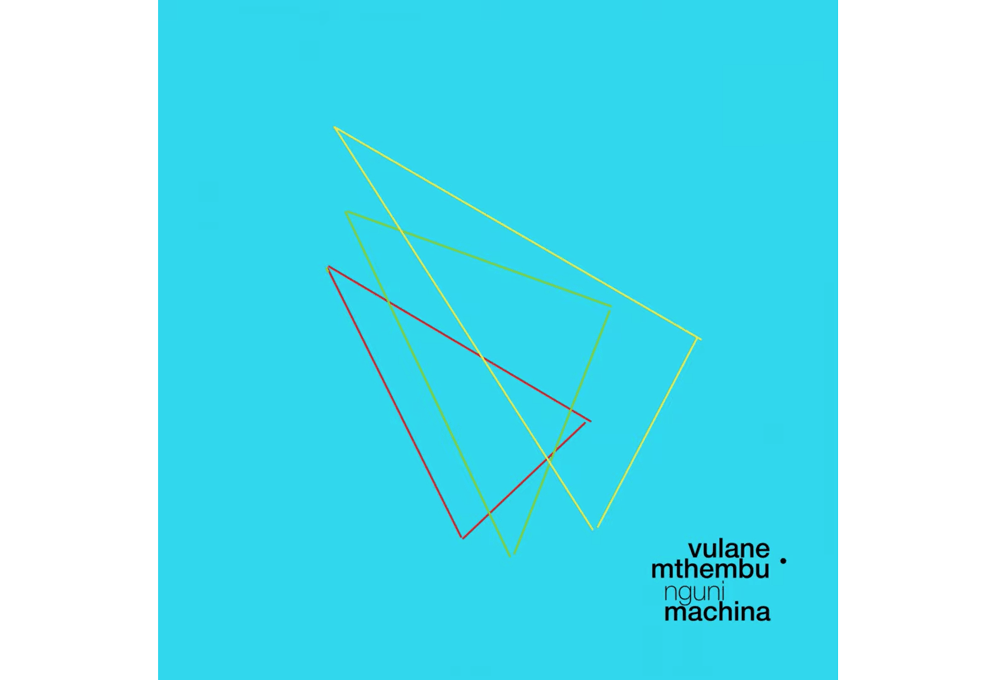
While working on a recent art project Nguni Machina which consisted of AI-produced music, I came across such barriers as most open-source datasets were based on Western music and this is not unique to music and is indeed prevalent in all artforms and spaces.
Democratization of data is absolutely necessary to achieve a more complete AI which is void of cognitive bias imbued by the creator and we are not at this stage yet.
AI can either reduce or reinforce societal stratification if the data is skewed and inaccurate. Despite Africa being at the forefront of this revolution in terms of innovation and current work done in this field, we are still lagging far behind in terms of datasets. This makes it hard to create competent AI systems which speaks to the African experience. This leads to various ethically questionable implementations which sustain gender, racial, class and cultural biases. The biggest challenge to AI reaching its full potential is a diversity problem.
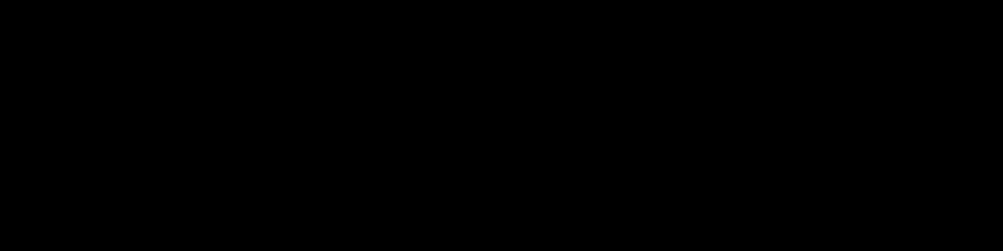
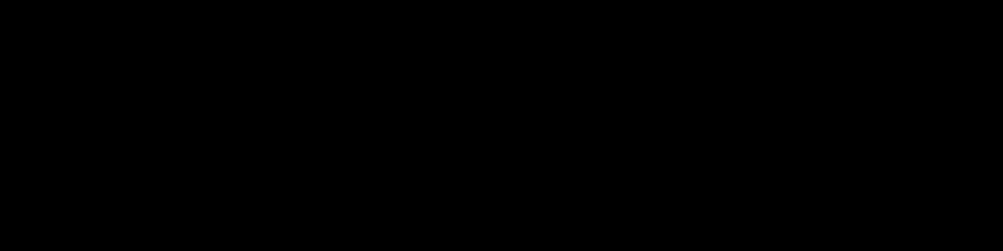
There are of course many African-based projects working to address this problem but there aren’t enough of them and the technology is evolving at an incredibly fast pace for them to catch up.
AI will inevitably take over the majority of tasks in our lives and has already proven to be even more adept and competent at many tasks than us humans. It is therefore important that we actively engage with our offspring. That we acknowledge that we are the ancestors in our lifetime and we pass on the requisite indigenous knowledge systems, culture and values. As an open-data advocate I feel this will be the fastest way for Africa to catch up and offset the heavily westernised dataset landscape that already exists, by freely sharing and enabling access for everyone to use African data in their models we will enable accelerated adoption and ensure more robust machine learning deployment.
